Possibilities and Prospects of Using Generative AI in Manufacturing
It is important to note that in recent decades the industrial scene has changed drastically due to achievement of artificial intelligence technology. Of all these innovations, generative AI can be said to be the one that holds the prospect of turning the manufacturing industry on its head. While in the traditional AI technology, information is formalized into pre-defined rules and datasets, generative AI is a type of deep learning models to create new content, designs, and solutions from scratch. This capability is more proficient especially in manufacturing since they require innovation, efficiency, as well as accuracy.
Generative AI can analyze a huge amount of data to uncover patterns and insights that were previously unattainable. To manufacturing professionals, this means more than static optimisation; it is the progression towards intelligent manufacturing. And as the overall challenges of the industry are rising costs, lack of qualified personnel, increased demand for customization, generative AI is presented as a kind of savior for the industry.
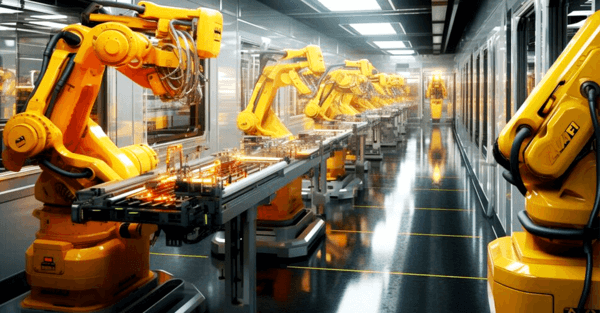
Optimizing Production Workflows and Processes
One of the most significant advantages of generative AI in manufacturing is its ability to optimize production workflows and processes. Such approaches have been known to be time-consuming as they involve a lot of testing and are thus uneconomical. While there are instances where generative AI models are incapable of implementing production optimisation, it can at least model several production possibilities and determine the best methods.
Generative AI can take data coming from sensors, machines, and historical production records, and can predict bottlenecks, suggest process improvements, and even automate decision-making processes. For example, restrictive features of scheduling can be enhanced using AI algorithms where factors including machine availability, workforce capacity, and material supply. This results in minimization of idle working time, increased capacity of work, and in overall production expense, lower costs.
Besides, generative AI can facilitate real-time adjustments. In dynamic manufacturing environments, conditions could be dynamic and need attention which requires action. With the help of AI it is possible to get constant supervision of the processes taking place within an organization, and appropriate correction in real time. This adaptability not only enhances efficiency but also ensures that production goals are met consistently.
Improving Quality Assurance and Reducing Defects
Quality assurance is always a critical concern in manufacturing, as their failure can lead to significant financial losses and damage to brand reputation. Generative AI provide the best way of improving quality assurance processes and reduce defects.
Typically, quality control involves manual inspections and audits in addition to spot checks, which might be time-consuming besides being susceptible to human error. While generative AI can be used to review certain images, signals and other inputs from sensors in order to identify possible defects and discrepancies. It means that, using machine learning techniques, one can train the system to detect even minor deviations from the standard and pinpoint the defects that will inevitably emerge during the production of new items and their distribution in the market.
Not only can generative AI be applied for detection, root cause analysis can also be performed by the use of generative AI. Through pattern and correlation analysis AI systems can determine the actual reasons of getting defects, so manufacturers can take measures to eliminate these issues in advance. This not only helps minimize the incidence of defects within the product but also assists in enhancing the rehabilitation of the manufacturing process.
Enhancing Predictive Maintenance and Asset Management
General maintenance and asset management are critical areas where generative AI can make a significant contribution. Traditional maintenance strategies include the reactive maintenance and preventive maintenance which are frequently associated with either high levels of system unavailability or frequent maintenance intervention. The generative AI application of predictive maintenance provides a more proactive and effective solution.
Through big data analysis, generative AI can predict when it is time for maintenance based on data from equipment sensors, maintenance history, operating logs among others. This predictive ability makes it easier for manufacturers because they are able to deal with problems that may be likely to cause equipment failure, thereby minimizing downtime and reducing maintenance costs.
In addition, generative AI can help the organization enhance the efficiency of asset management by offering an understanding of the usage and wear and tear of the machinery and equipment owned by the firm. Through analysing the frequency and severity of wear and tear, operational managers can justify decisions on the timing of replacement and additions to the equipment systems. This early engagement also guarantees that the assets are exploited optimally, improving the general operation efficiency.
Accelerating Product Design and Innovation
Manufacturing is fostered by innovations, and generative AI is a catalyst for accelerating product design and development. The activities that are historically associated with designing a product may take a lot of time and resources to complete, and a number of cycles of prototyping, testing, etc. Generative AI can streamline this process by generating and evaluating numerous design alternatives in a fraction of the time.
AI-driven design tools can integrate big data sources to put forward new innovative solutions that satisfy certain conditions like lighter structures, less material usage, and cheaper production. The mentioned tools can also take into account constraints and preferences set by engineers while generating design, and as such, the result will be feasible and creative.
Also, generative AI could help to integrate several design teams in that they would be working in one environment and using one platform for presenting and discussing ideas. This kind of setting enhances the rate of iteration helping organizations to bring new products to the market faster. Generally, generative AI makes efficiency, time, and costs of developing and bringing products to the market less of a challenge thus making manufacturers remain relevant in the ever-shifting market.
Conclusion: Unlocking the Full Potential of Generative AI in Manufacturing
AI integration in manufacturing has the prospect of the potential to respond to several of the industry’s major challenges. Started with reducing production time, improving product quality, increasing effectiveness of maintenance planning, and increasing accelerating product design, generative AI offers a comprehensive suite of solutions that drive efficiency, innovation, and competitiveness.
Altogether, for SaaS professionals in the USA, the implications of the reveals listed above are, indeed, profound. What’s more, the SaaS providers can use generative AI for giving manufacturing businesses unique and superior resources that can promote efficiency and innovation. Thus, the ability to integrate AI-driven solutions without disturbing existing structures will become a significant competitive advantage in the rapidly expanding market of intelligent, data-based applications.
Today, it becomes easier to identify specific technologies that are going to bring the desired results in the sphere of manufacturing industry and generative AI seems to be the key one in this case. Thus, manufacturers adopting it can not only solve the present challenges also position themselves for sustained success in the future.
Author bio:
Antje Fried is a passionate tech enthusiast and accomplished content writer at Helios Solutions. With a keen interest in AI advancements, she specializes in crafting insightful articles about cutting-edge tools like SolBI AI. Her work bridges the gap between complex technology and everyday users, making innovation accessible and engaging.